Asaf Nadler 🗣 | Jordan Garzon 🗣
Abstract (click to view)
In this talk, we present a system to identify and track unsafe services that are hosted on bots. The system operates by identifying services whose hosting IP address was marked as a bot by an IP reputation threat intelligence due to engaging in cyber attacks (e.g., D-DoS), and that the hosting IP is not shared with other web services. The system was implemented using Akamai’s IP reputation system that interacts with over 1.3 billion devices on a daily basis, and identify bots if they issue cyber attacks against websites that are hosted on the Akamai CDN platform which serves up to 30% of the world’s entire web content. Among others we focus on machines involved in D-DoS attacks, SQL injections and account takeovers campaigns. After acquiring the IP address of the bots, we scan over 2.2 billion daily DNS queries that go through the Akamai platform to identify domains that are uniquely resolved to the bots’ IPs and mark these domains as unsafe for use. The system results in thousands of unsafe domains on a weekly basis that are constantly tracked for analysis and active protection.
Jordan Garzon 🗣 | Asaf Nadler 🗣
Abstract (click to view)
The source code of botnets is often leaked online and re-used by new botnets. The re-use of source code assists bot-owners in quickly setting up their botnets, but it also inherits similarities to known botnets that can assist in detection. Most specifically, the URL paths that a bot uses to communicate with their C&C are often re-used.
In this talk, we present a system to identify patterns in URL paths that serve known botnets in order to block them if they are ever re-used by new botnets. The results of the systems are intended for use in an inline, high-performing HTTP proxy and accordingly, existing solutions that target malicious URL detection such as neural networks are inconsiderable. Instead, we construct an offline language model using the Smith-Waterman algorithm, cluster it and use a known set of genetic algorithms to propose regular expressions that match on sets of bot C&C URLs without matching any benign URL. Our experimental setup includes 1.4M URLs, both bot C&C and benign, and our initial results yielded 1.3k new bot C&C URLs, and a 96.3% accuracy for patterns that appeared at least twelve times within the training data. Moreover, the system is currently being deployed on a large-scale HTTP traffic to report results over time.
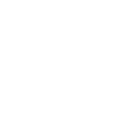